Artificial Intelligence and Machine Learning algorithms are certainly two double-edged swords. Alongside being the two of the most promising and immensely popular technologies, they also manage to portray the darker reflection of today’s somber society. Every day the gory and dark image is getting even bloodier, and things are getting out of hand akin to a scarily realistic sci-fi web series. Deepfake is one of the most significant examples out there.
What is Deepfake?
Driven by the new generation of generative deep neural networks, which is capable of synthesizing videos from a database of training data with least manual editing, Deepfake can effectively create unbelievably real videos using a single photograph of the target. The outcome of this AI-driven technology is incredibly convincing, and thousands of fake-porn videos flooding the internet is just the tip of the iceberg.
The name deepfake originates from a Reddit user named “deepfakes” who purportedly handled a community dubbed r/deepfakes and circulated numerous videos carrying facial images of celebrities and the bodies of the pornographic actresses. The almost real-looking videos caught the attention of mainstream media, and, Reddit banned the user. However, the technology evolved and was under the spotlight in June 2019 when Vice.com reported that artist Bill Posters in association with Daniel Howe had posted four impersonated high-quality videos of Donald Trump, Mark Zuckerberg, Morgan Freeman, and Kim Kardashian.
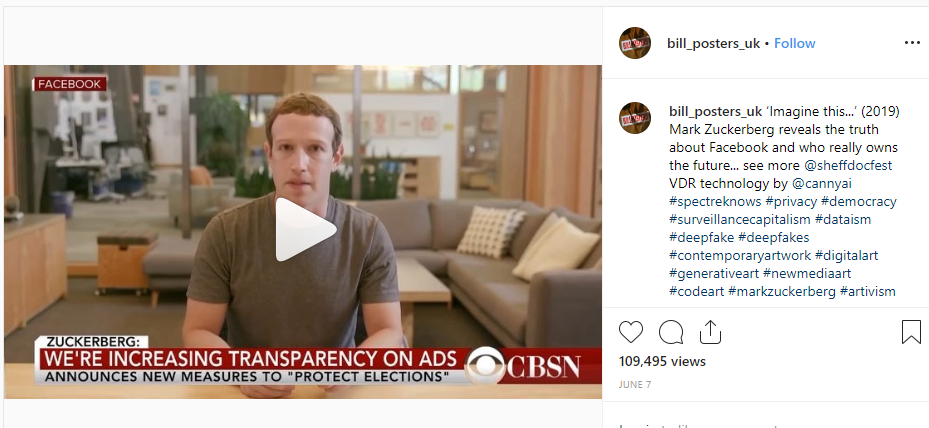
A deepfake of Facebook boss Mark Zuckerberg was also posted in Instagram
Weeks later, researchers at Samsung’s AI lab in Moscow demonstrated how powerful the Neural Network powered AI imagery has evolved into by posting a few “living portraits” of Leonardo da Vinci’s famous painting Monalisa, legendary Russian author Fyodor Dostoevsky, Hollywood legend Marilyn Monroe and surreal artist Salvador Dali. These eerily realistic talking head models were created using convolutional neural networks and a single image of those celebrities.
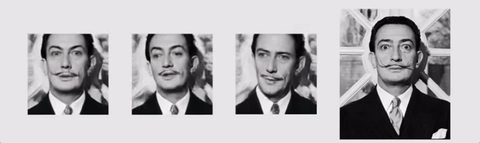
Figure 1 Puppeteering results for one-shot models learned from photographs in the source column.
To get the visage for those living portraits, the researchers used numerous publicly available human videos from Voxceleb database and mimicked the unique human face features such as eye movement, mouth shape, the length and shape of the nose bridge.
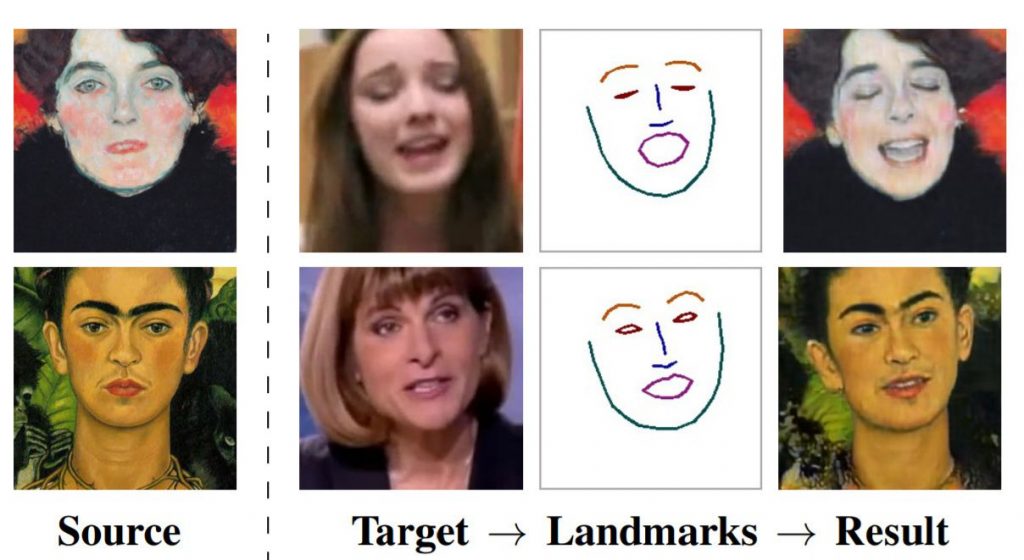
Figure 2 The results of talking head image synthesis using face landmark tracks extracted from a different video sequence of the same person (on the left), and using face landmarks of a different person (on the right).
Though Samsung researchers have claimed that this technology will help develop realistic face-avatars for video conferencing, gaming and special effects videos, the finding holds the potential to empower other generative adversarial networks (GAN) powered algorithms such as Deepfake.
Samsung AI’s research has taken a big leap forward by reducing the requirement of numerous images of the target, and in the future, the technology could pose a significant threat to the lives of ordinary people.
Demystifying Deepfake
Deepfake videos use dual AI systems – generator and discriminator. The generator is responsible for creating the video clip, while the discriminator determines the generated clip’s authenticity. Each time the generator creates a video clip, the discriminator learns the pitfalls in the resulting video to ensure those mistakes aren’t repeated in the subsequent operation. Together, the two methods create a technology known as Generative Adversarial Network, aka GAN. Each time the AI creates a video, GAN identifies the output and creates a training dataset for the generator.
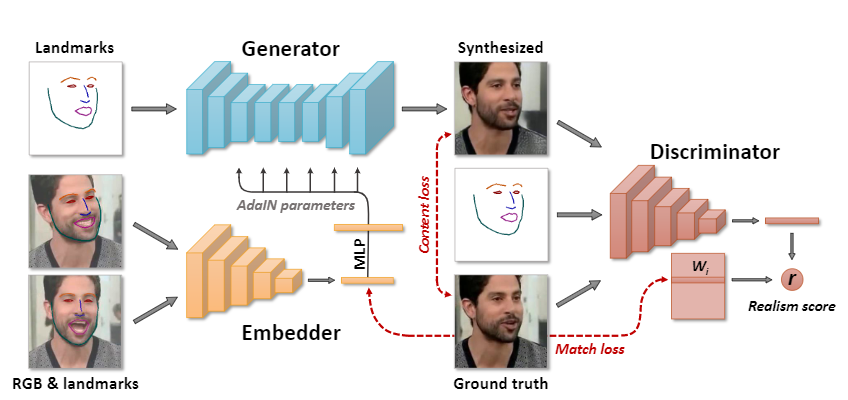
Figure 3 The meta-learning architecture involves the embedder network that maps head images (with estimated face land-marks) to the embedding vectors, which contain pose-independent information.
The latest discovery by Samsung AI Labs will help the GAN feed massive datasets to the system, and, by using the inputs, the system will try and become stronger every day as neural networks do. For instance, OpenAI’s GPT-2 used 40GB worth of internet text as data set and produced a written language so well articulated that caused fear in its developer for the potential it holds in helping fake news production. The developer decided to cease its release.
The downside to this technology is, all though it requires a single image of the victim to create a video, the system will also need a massive data set for training the model, and, hence will demand tremendous system resource and time.
However, anonymous developers are releasing easy-to-use web tools by offering such sophisticated technologies to help novice users. For example, an anonymous developer reportedly released software on June 20, 2019, dubbed ‘DeepNude.’ The online app can convert any women’s picture to a naked one. Initially launched for Windows and Linux machines, the neural network based downloadable application doesn’t require any expertise to operate. The resultant images come with a giant watermark which could be removed by purchasing the paid version for a measly US$ 50.
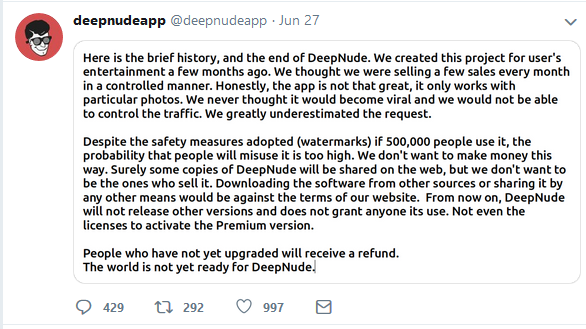
Figure 4 Deepnude has been taken down but it still available on the internet
Following the media coverage, the developer took down the app from the internet. Not surprisingly, the source code of the app started spreading over the Internet, and some developers even tried to reverse engineer the source code to reincarnate the app from its grave.
Looming threat on the Enterprises
Concern about technologies such as Deepfake is not limited to individuals or celebrities. They hold the potential to disrupt business enterprises as well. Alongside the damage to institutional reputation, these technologies could expose security vulnerabilities, which will be challenging to combat. Attackers can create realistic fake videos or audio tapes to harm the brand image and use social media tools to damage or destroy them in public eyes. It can also help to create fake digital identities and launch more disruptive phishing attacks on enterprises.
The risk extends to fake videos of politicians, civil executives, armies, police, and celebrities to damage their public image. Indeed, a scary thought.
Detecting Deepfakes
Fake videos produced using Deepfake is not easily identifiable as counterfeit and thus became an acute challenge for digital forensics. Using GAN, Deepfake videos are impersonating the targets effectively, becoming hard to detect unless one seeks the help of experts. The AI makes the videos so realistic that even traditional media forensics fail to recognize such videos as fake ones.
However, the latest research claims that fake videos can be identified by tracking physiological signals intrinsic to human beings and aren’t captured by the data sets used for making such synthesized videos. For instance, the researchers mentioned few spontaneous and involuntary physiological activities such as breathing, pulse, and eye movement, often ignored in such videos.
The research work focused on the regularities of detection of eye blinking through a novel deep learning model combining a Convolutional Neural Network (CNN) with a Recursive Neural Network (RNN).
Human eye blinking is classified into three types – spontaneous, reflex, and voluntary. The spontaneous blinking happens without any external stimulation and internal effort and serves an essential biological function moisturizing with tears and removing irritants from the surface of the cornea and conjunctiva.
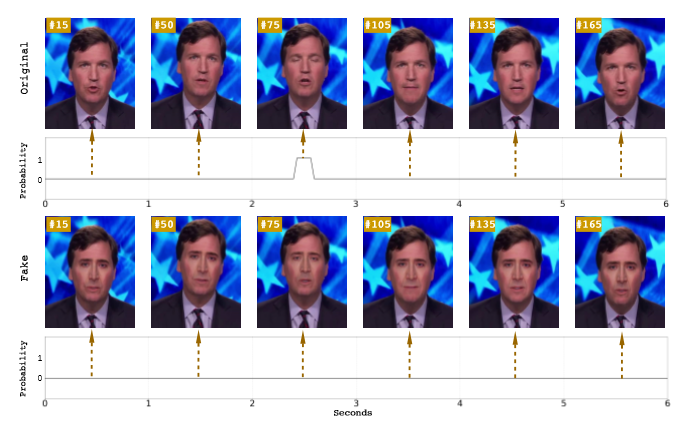
Figure 5 Example of eye blinking detection on an original video (top) and a DeepFake generated fake video (bottom. Note that in the former, an eye blinking can be detected within 6 seconds.
The researchers further explained that spontaneous blinking rate is usually 17 per minute or 0.283 blinks per second, which increases up to 26 blinks per minute during any conversation and decreases to 4.5 per minute while reading.
Interestingly, AI-generated faces usually lack the essential eye blinking function because most training datasets refrain from featuring face data with eyelids closed.
The research paper further illustrates how they locate the particular face area using a face detector and checks each frame of the video to check its authenticity. Alongside eye blinking, they also detect key face areas, including the tip of the eyes, nose bridge, and the contours of the mouth.
The detection technology sounds quite convincing, and it might be possible to detect numerous fake videos using these methods. However, Deepfake developers will soon train the neural network to come up with more convincing ways to override these detection process and threat more individuals and enterprises.
To be safe from such lethal threats, government authorities and enterprises should come forward and begin an awareness campaign to help people to identify fake videos. The authorities should also create guidelines to help people when they fall victim to the clutches of those who fail to understand basic human decency.